
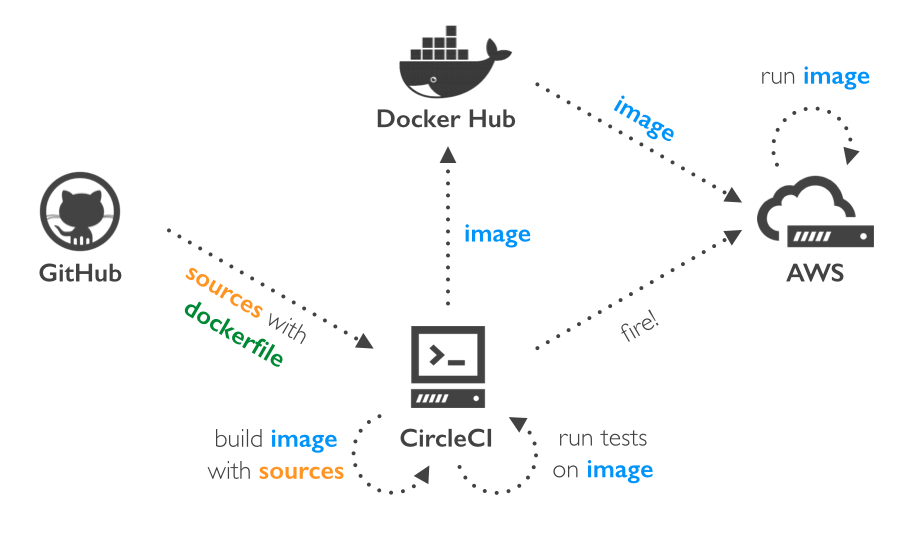
They face an abrasive development workflow that drags down their velocity.They confront lose-lose choices when dealing with environments and dependency management.They struggle to understand whether data is up-to-date and to distinguish trustworthy, maintained data from one-off artifacts that went stale months ago.They constantly catch errors in production and find that deploying changes to data feels dangerous and irreversible.It executes pipelines in production, but makes it hard to work with them in local development, unit tests, CI, code review, and debugging.ĭata teams who use Airflow, including the teams we’ve previously worked on, face a set of struggles: It schedules tasks, but doesn’t understand that tasks are built to produce and maintain data assets. Airflow’s design, a product of an era when software engineering principles hadn’t yet permeated the world of data, misses out on the bigger picture of what modern data teams are trying to accomplish. Airflow dutifully executes tasks in the right order, but does a poor job of supporting the broader activity of building and running data pipelines. 2023), Sandy Ryza provides a detailed comparison of Airflow and Dagster.ĭata practitioners use orchestrators to build and run data pipelines: graphs of computations that consume and produce data assets, such as tables, files, and machine learning models.Īpache Airflow, which gained popularity as the first Python-based orchestrator to have a web interface, has become the most commonly used tool for executing data pipelines.īut first is not always best.
Airflow docker run parameters update#
In an update to this article's content (Feb.
